A wave of recent success stories has ushered AI into the mainstream, prompting (no pun intended) many to imagine possibilities that previously felt out of reach. The heyday of Large Language Models (LLMs) is here, and as ChatGPT has shown us, AI applications are now capable of drafting marketing emails, writing code and ordering our groceries. The pace of innovation is accelerating and staggering, both in terms of new models and build-on-top applications. It’s clear even to those not tapped into the AI world that things are heating up – from the release of ChatGPT in November, to robots that operate on LLMs in March (Google PaLM-E), and AI systems delegating to AI systems (AutoGPT) just a few weeks later. Everyone is now asking themselves what it all means for the way we work. In particular, one recent development has caught our eyes at Proscia: Meta’s Segment Anything Model (SAM).
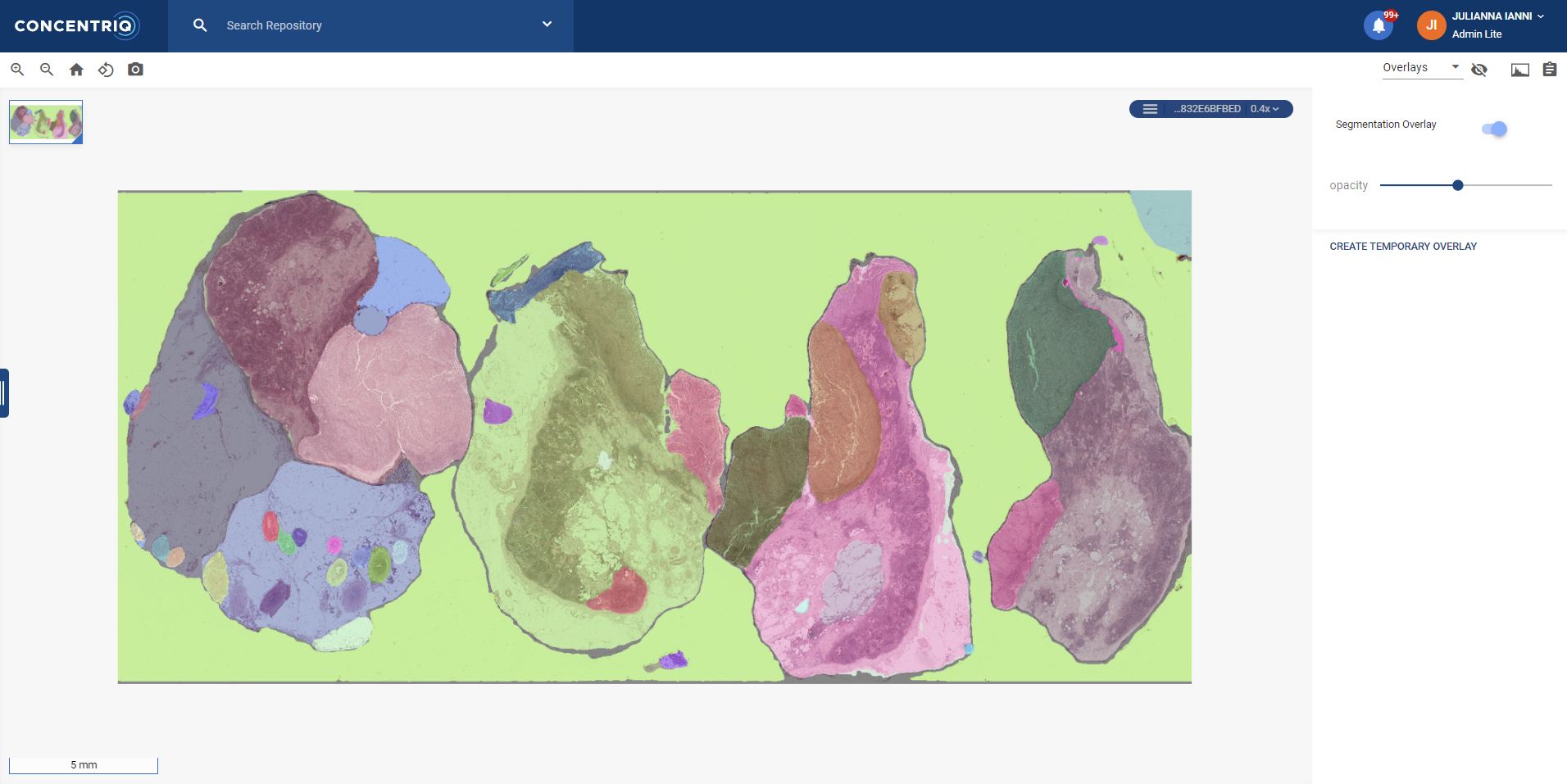
SAM is a model trained to identify, “cut out” or “annotate” areas of interest within an image – typically referred to as segmentation. There are many, many models that do segmentation, so that in and of itself isn’t special. But what is quite remarkable is essentially zero-shot generalization: SAM can segment images well, even when it hasn’t seen anything similar before.
In the context of digital pathology, technologies like SAM are game-changers. One of the major factors affecting the development of AI applications today is data. Until very recently, most models or applications that came out for typical images (think: photographs, etc.) have been completely unsuitable for pathology applications. They just didn’t work. In the past, it would have taken months to years, tens of thousands of images and professional annotations, and hundreds of thousands of dollars to build a segmentation model like this. In contrast, my team at Proscia was able to build a proof of concept integration of SAM with our digital pathology platform Concentriq quickly.
Within a matter of hours, SAM was out of the box and working, or pretty close to working, to highlight regions of interest in pathology data. Now, what constitutes “working” may differ a lot by task, e.g. whether you want to segment tissue or identify a specific kind of tumor or outline all the nuclei in an image. So importantly, the other innovation that’s enabling for SAM is “prompting” – which you may have heard of in relation to ChatGPT – but in this context, means the series of user inputs (think: single mouse clicks or bounding boxes) that a user can use to tell SAM their intent. (There’s a nice primer by Andrew Ng here.)
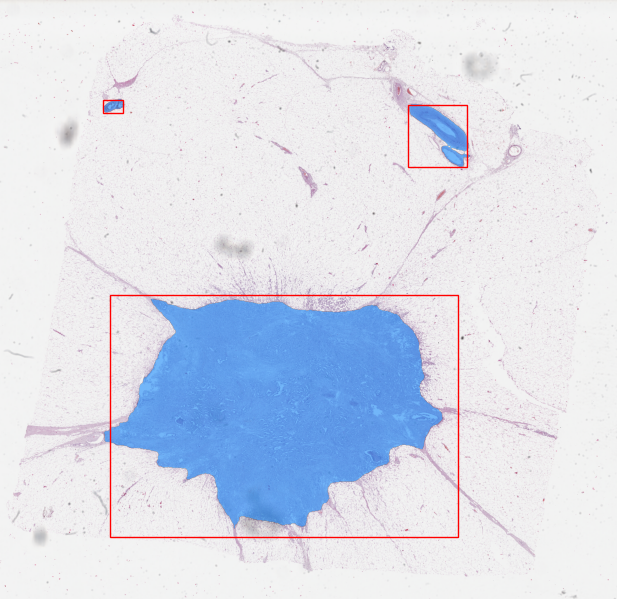
In pathology, you might use prompts in the form of clicking on cells you want annotated (positive annotation) and cells that you don’t (negative annotation) to tell SAM or another AI system you want inflammatory cells segmented but not other types of cells. Clicking on a few nuclei, for example, and a few extra clicks to tell it that stroma etc. is background, generates a fairly good nuclei segmentation. This is something that could take quite a bit of time to do with traditional tools. The SAM developers even show an example of using text input as a prompt. Imagine just typing in “inflammatory cells,” and they are annotated for you! It feels a bit like science fiction, but these are some of the real possibilities we can envision with innovations like SAM and LLMs. SAM, incredibly, comes within shouting distance of state of the art methods specific to individual tasks in pathology. Will it replace any of them tomorrow? Depending on the task, maybe and maybe not. But the idea that we can get this far with a model that has never seen pathology data is truly amazing.
Beyond SAM
SAM is just one of the latest breakthroughs that has us excited about AI applications in pathology. Most of the buzz outside pathology today, though, is about LLMs, and even the idea of prompting SAM with text gives us a glimpse of where the field is headed. It’s becoming clear that language is the language of AI. For years, computer vision and language models were two distinct branches of AI. Now, we see them merging, opening many possibilities; your computer vision applications are going to speak the same language that you do.
Take, for example, Google’s PaLM-E 2. It not only uses language to ask a robot to perform tasks but also sets a new state of the art for visual question answering (OK-VQA). By training on image and language data, you can quite literally chat with your images. When you ask it how it can be helpful and show it a picture of a dirty table and chairs, for example, it can tell you that it can be helpful by removing the trash from the table, etc. It’s almost mind-blowing to think of what we can do when the same technology is applied to pathology images. Have you chatted with your borderline melanoma cases lately? What would you ask them? Maybe I would ask my AI if it would order PRAME on this case, or what my expert colleague down the hall would say about it? Maybe I would just tell it to send the case down the hall for me. Maybe I would ask it to give me more cases like this one so I can perform a study on them. There are literally more uses than anyone can think of.
Of course, it’s one thing to dream up a use case or to toy with a demo in your web browser, and another to have an AI application running in production in your digital pathology platform. And we’re not here to say that you’re missing out if you don’t go run out and try to get SAM or ChatGPT in your lab tomorrow. We’re more excited about the possibility and promise of today’s most groundbreaking technologies when brought to pathology. The pace of innovation over the past few months has given us a taste of what’s to come. A new era of AI-enabled pathology is approaching faster than almost anyone believed it would. Tomorrow’s “SAMs” will change the way we practice and give us the tools to work better – and Proscia will be ready.