The advent of Whole Slide Imaging (WSI) has enabled a major transformation in pathology, enabling clinicians to rapidly digitize pathology slides inexpensively into high resolution images. With slides now being routinely digitized, the revolution of deep learning methods in computer vision can be brought to large imaging datasets and used to build tools that could help improve the efficiency and effectiveness of pathology departments, clinicians, and researchers in diagnosing and treating cancer.
Consider the most prevalent cancer in America: skin cancer. Each year more people in the United States are diagnosed with skin cancer than all other cancers combined. Overwhelmingly, the most life-threatening skin cancer is metastatic malignant melanoma, for which the 5-year survival rate of patients is less than 20%. A Pathology Deep Learning System (PDLS) that prioritizes melanoma cases could improve turnaround time, which is particularly important for melanoma as turnaround time is correlated with overall survival. However, diagnosing melanoma is extraordinarily challenging and there is a high rate of disagreement among pathologists of what qualifies as melanoma, making accurate melanoma detection a particularly relevant and challenging problem to solve. One study even quantified this rate of disagreement to be 40%.
In our submission to the CDPath workshop at ICCV, we describe a PDLS, currently for research use only, that can classify skin cases for triage and prioritization prior to pathologist review. Our PDLS enables melanoma prioritization by performing hierarchical classification of skin biopsies into one of several classes, including classification of “Melanocytic Suspect,” representing likely melanoma. Based on published literature to date, our PDLS is the first to classify skin biopsies at the specimen level rather than the slide level. After removal, a tissue biopsy (specimen) gets chemically processed, thinly sectioned (sliced), placed onto glass slides, and stained. A single biopsy could result in multiple slides, which when scanned become Whole Slide Images (WSIs). By performing classification at the specimen level directly (not classifying individual patches or slides), our Deep Learning system leverages the full collection of WSIs that represent the entirety of the tissue to perform a classification, modeling the process of a dermatopathologist who reviews the full collection of scanned WSIs to make a diagnosis.
Obtaining reliable annotated data is always a challenge in developing a deep learning model. When the “ground truth” varies from one pathologist to another, it’s difficult to train a model, but also impossible to report results reliably. To address the high rate of melanoma disagreement among pathologists, we trained the PDLS from a single dermatopathology lab (called the Reference Lab) that is a top academic medical center (Department of Dermatology at the University of Florida College of Medicine) on melanocytic specimens where the diagnosis was agreed upon by multiple dermatopathologists. This dataset is the largest consensus melanoma dataset compiled to date. We also tested the model on two additional top academic medical centers (Jefferson Dermatopathology Center, Department of Dermatology Cutaneous Biology, Thomas Jefferson University, which we call Validation Lab 1, and Department of Pathology and Laboratory Medicine at Cedars-Sinai Medical Center, which we call Validation Lab 2).
Pathology Deep Learning System Architecture
Our PDLS is composed of three main stages: a quality control stage, a feature extraction stage, and a hierarchical classification stage. We only keep tissue-containing regions in the WSIs that comprise a specimen, and further subdivide each WSI into 128×128 pixel tiles. Each tile was passed through the quality control and embedding components of the PDLS. The quality control stage consists of ink filtering, blur filtering, and image adaptation and was designed to ensure high quality tiles were used in model training. The feature extraction stage captures higher-level features in these tiles by propagating them through a ResNet50 neural network that was pretrained on the ImageNet dataset to embed each input tile into 1024 channel vectors.
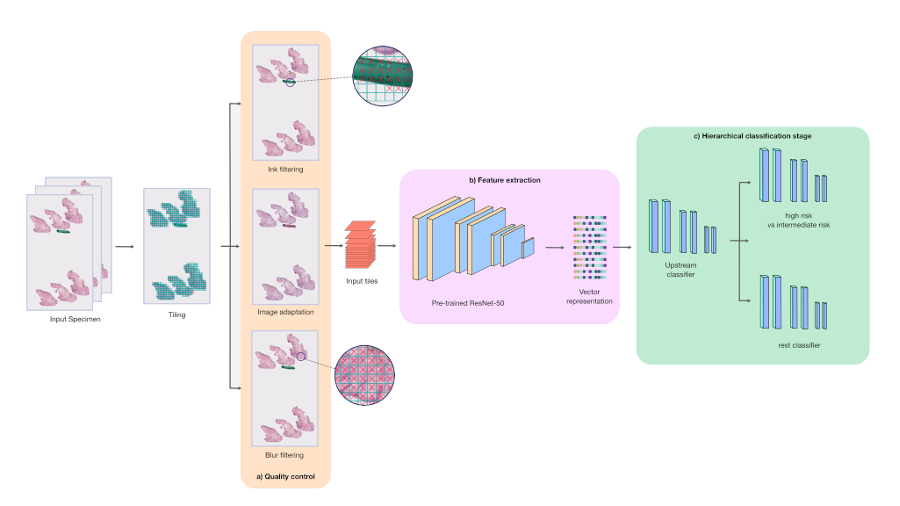
The hierarchical classification stage classifies specimens into one of six classes; however, we focus our discussion here on the “Melanocytic Suspect” class, which combines two classes:
- Melanocytic Intermediate Risk: Severely atypical melanocytic nevi (suspicious mole or skin growth) or melanoma in situ
- Melanocytic High Risk: invasive melanoma
The three models in the hierarchy each consisted of four fully-connected layers and were trained under a weakly-supervised multiple instance learning (MIL) paradigm. The hierarchical classification stage was designed in order to classify Melanocytic Suspect specimens with high sensitivity. First, an upstream model performed a binary classification between Melanocytic Suspect specimens and the other four classes in our model (which we collectively define as the “Rest” class). Specimens that were classified as “Melanocytic Suspect” were fed into a downstream model, which further classified the specimen between “Melanocytic High Risk” and “Melanocytic Intermediate Risk”. The remaining specimens, classified as “Rest”, were fed into a separate downstream model, which further classified the specimen among the remaining four classes, which includes two more common forms of cancer called basal and squamous cell carcinoma. The hierarchical model architecture of the PDLS provides pathologists flexibility to distribute their workloads in order to prioritize cases they’re most comfortable diagnosing.
Performance
Although our PDLS classifies specimens into one of six classes, we focus our discussion on the upstream Melanocytic Suspect vs Rest task, which draws the dividing line between likely melanoma and other skin specimens. The Receiver Operating Characteristic (ROC) curves are shown below, where the Area Underneath the ROC Curve (AUC) values were found to be 0.94 & 0.93 for the Melanocytic Suspect and Rest classes, respectively, for the Reference Lab, 0.95 & 0.96 for Validation Lab 1, and 0.82 & 0.82 for Validation Lab 2.
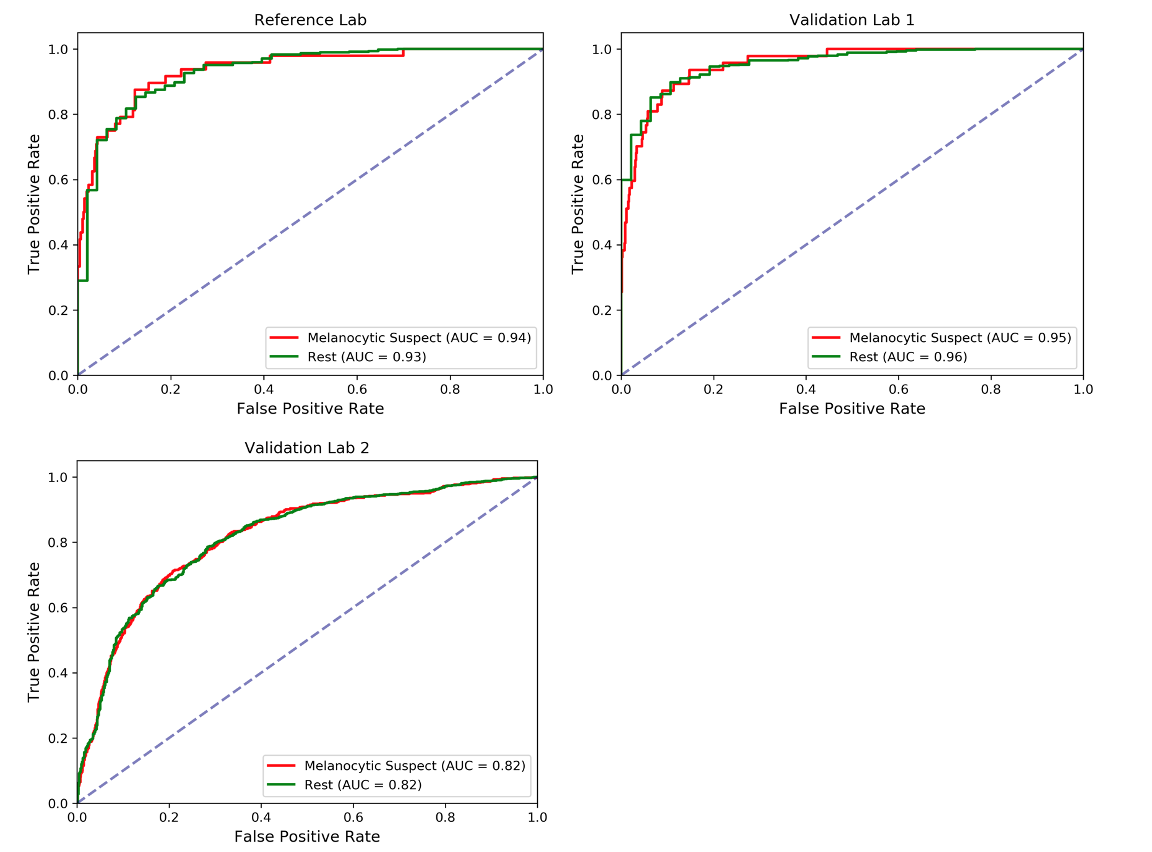
This high performance to the Melanocytic Suspect class enables automatically sorting and triaging skin specimens prior to review by a pathologist. Existing methods provide diagnostically-relevant information on a potential melanoma specimen only after a pathologist has reviewed the specimen and classified it as a Melanocytic Suspect lesion, and often take days to deliver results. The PDLS’s ability to classify suspected melanoma prior to pathologist review could substantially reduce diagnostic turnaround time for melanoma by not only allowing timely review and expediting the ordering of additional tests, but also ensuring that suspected melanoma cases are routed directly to subspecialists, with a classification that only takes several minutes. The potential clinical impact of a PDLS with these capabilities is underscored by the fact that early melanoma detection is correlated with improved patient outcomes. To demonstrate such a potential clinical impact, we envisioned a scenario in which a pathologist’s worklist of specimens is sorted by the system’s confidence (in descending order) in the upstream classifier’s Melanocytic Suspect classification. The figure below demonstrates the resulting sensitivity to the Melanocytic Suspect class against the percentage of total specimens that a pathologist would have to review in order to achieve that sensitivity. In this dataset, we show that a pathologist would only need between 30% and 60% of the caseload to address all melanoma specimens.
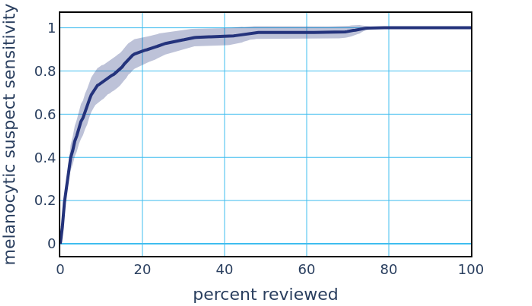
We have shown that our PDLS is highly sensitive to melanoma, is capable of automatically sorting skin specimens, and is built to be real-world ready without requiring pixel-level annotations. Additionally, by training on consensus of multiple dermatopathologists, our PDLS may have the unique ability to learn a more consistent feature representation of melanoma and aid in flagging misdiagnosis.
Acknowledgements
We would like to thank the support of Jeff Baatz and Liren Zhu at Proscia for their engineering support; Theresa Feeser, Pratik Patel, and Aysegul Ergin Sutcu at Proscia for their data acquisition and Q&A support; and Dr. Curtis Thompson at CTA and Dr. David Terrano at Bethesda Dermatology Laboratory for their consensus annotation support.